Systems-Level Modeling
of Defect Formation in Silicon (and Silicon-Alloy) Materials Processing
The silicon (and to a lesser extent, silicon alloy)
crystal growth and wafer processing industry continues to generate
a large experimental database characterizing the properties of numerous
defects and impurities that plague microelectronic devices. This
extensive database is tremendously useful for multiscale model development
and testing because the defects of current interest (voids, dislocation
loops, impurity complexes) are nanoscale features that are spatially
distributed over macroscopic length scales, i.e. in wafers and crystals.
It is also notable that many of the microscopic mechanisms responsible
for defect generation and evolution are generally important for
understanding a broad range of phenomena. Our work in this area
therefore is motivated by two complementary needs:
(a) The need for detailed quantitative experimental
data to test our models, mechanisms, and theories of microstructural
evolution in solids.
(b) The need for predictive models by industry
to optimize their processes for semiconductor wafer fabrication,
especially in the face of dramatically increasing development
costs.
An important aspect of the experimental database
is its heterogeneity, i.e. there are many different types of measurable
data available from different experiments. However, many of these
data (and therefore the models for predicting them) are connected
at the atomic level because they are all dependent on the same basic
diffusion, reaction, and aggregation of simple point defects such
as vacancies, interstitials, and impurities that are always present.
Despite the decades of research in this area, a major obstacle in
the development of robust and predictive process models for the
silicon crystal growth industry has been, and continues to be, the
uncertainty associated with the thermophysical properties of these
building blocks.
Our contributions in this area include the development
of a comprehensive suite of continuum models for various phenomena
including point defect dynamics during crystal growth, void formation,
metal diffusion in wafers, and oxide precipitate formation. However,
rather than consider each of these models and their associated parameters
separately, we have developed an inverse modeling framework that
allows for the simultaneous consideration of multiple phenomena
thereby taking advantage of the common microprocesses. As shown
below, this approach has two advantages over the traditional sequential
one: (a) the number of regression parameters is reduced, and (b)
the robustness of the parameters is increased because only a single
value for each one is allowed.
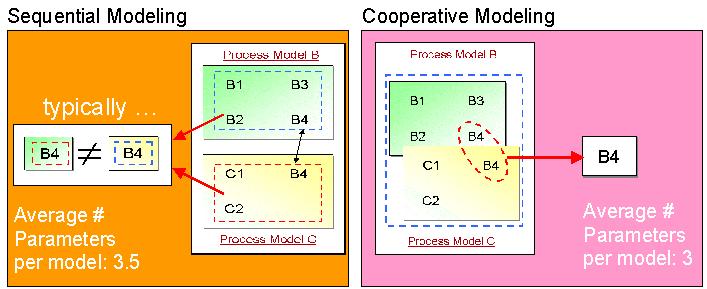
Figure 1: Cooperative approach
for multiple-model regression leads to fewer effective parameters
and greater exposure to experimental data.
|